The data analytics landscape continues to evolve. The first day of AWS re:Invent 2017 featured numerous breakout sessions covering the use of data lakes to support analytics. Some may dismiss the term as yet another buzzword, but Data Lakes are different.
From fintech to the gaming industry, organizations are discovering that Data Lakes provide lower storage cost and more flexibility than traditional Data Warehouses. Data Lakes also offer more flexibility, both in the data they can contain, and in the queries that can be run. Perhaps the greatest flexibility lies in the Data Lake’s capability to accept data from sources and in formats that were not anticipated at inception.
What is a Data Warehouse?
Traditional Data Warehouses store data, often using expensive database servers, using a rigid and carefully crafted schema. This allows certain reports and queries to be run very efficiently against large data sets.
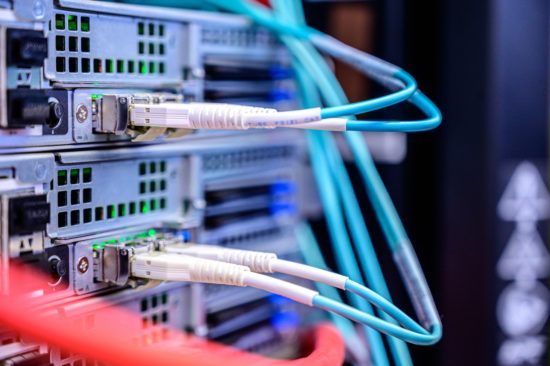
To achieve this homogeneity out of disparate data sources, complex transformations must be run. Aligning the data requires discarding some of the information and keeping just what is needed for the anticipated queries. So if you want to run a new query or drill down into the data in a new way, you may need to create a whole new set of transformations to run against the source data, if you even still have the data in its original format.
Diving into Data Lakes
The Data Lake strategy is to store the data as close to its native format as possible, and to impose no restrictions on the schema or even the format of the data. Instead of categorizing and transforming the data when it’s written to the repository, the data is transformed when it’s read. This strategy is made possible by the scalability and flexibility of cloud computing platforms.
A Data Lake is more than the mass of unstructured data — it is unstructured data positioned such that the computing power is readily available to transform and query the data. Since the repository contains the unmodified source data, no information is lost and any information contained in the data may be discovered. The tools for making these transformations are now mature and performant enough that they can be used at read time without requiring unreasonable amounts of time to run.
By using object storage like S3 or some hadoop variant, storage and related software licensing costs are greatly reduced when compared with enterprise scale database servers. Additionally these storage media generally have fault-tolerance built in, so backups and disaster recovery are no longer an issue.
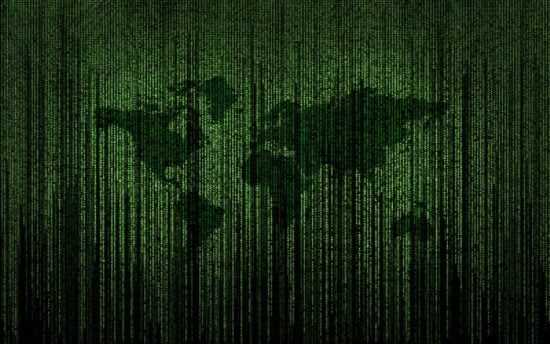
The strategy is not without drawbacks. The security for these systems is somewhat unproven. The biggest drawback is that the flexibility comes at the expense of ease of use. Transforming and querying data requires highly skilled data engineers and data scientists.
If you have large quantities of data, or if you need more flexibility in querying your data than your traditional BI tools can provide, maybe your data should be in a Lake.
Thanks for Reading!
Enjoyed this article? Check out some more of our recent, related articles in the area of data:
Data is the Oil & Analytics is the Refinery of the 21st Century
How Big Data Can Turn into Big Money
Which Cloud Platform is Right for You?